Machine Learning Could Improve Ability to Assess Effectiveness of Brain Disease Studies
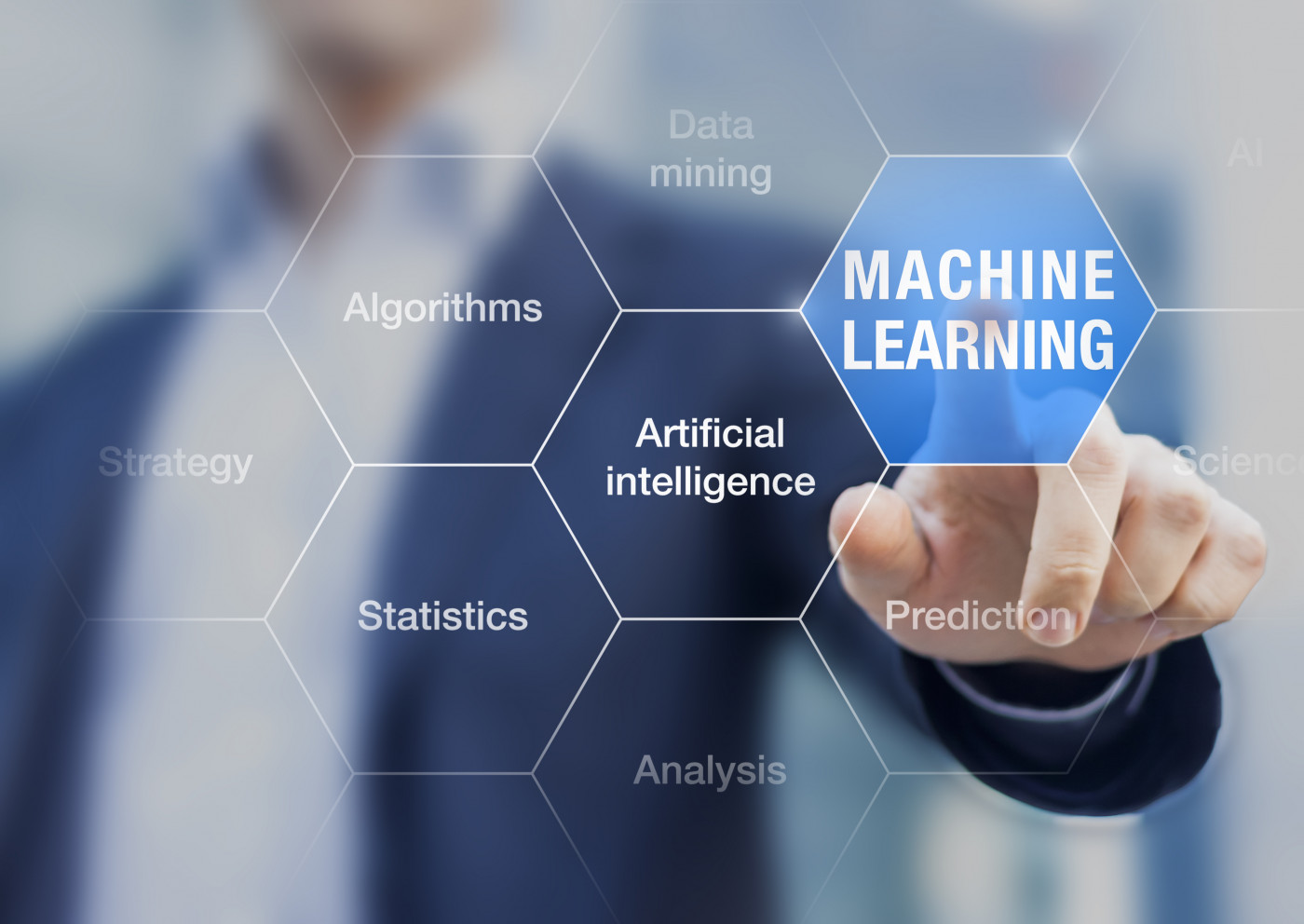
Machine learning methods could improve the ability of clinical trials to detect if treatments targeting the brain — such as for Angelman syndrome — are effective, as these methods are more sensitive to change than traditional statistical tests, say researchers from University College London.
Such methods could solve the problem of high variability among patients with a disease since they have the capacity to take into account thousands of variables and how they are linked to treatment outcomes — an impossible feat for traditional approaches.
The study backing up the claim, “High-dimensional therapeutic inference in the focally damaged human brain,” used brain damage caused by a stroke to examine the issue. But the same concept can be used for other brain diseases in which imaging or other objective data is available.
“Current statistical models are too simple. They fail to capture complex biological variations across people, discarding them as mere noise,” Parashkev Nachev, PhD, senior author of the study, published in the journal Brain, said in a press release.
“We suspected this could partly explain why so many drug trials work in simple animals but fail in the complex brains of humans. If so, machine learning, capable of modeling the human brain in its full complexity, may uncover treatment effects that would otherwise be missed,” he added.
To examine if their idea was correct, they gathered large-scale data from stroke patients. For each patient, they included the entire complex anatomical damage pattern. As a measure of how the stroke had impacted the brain, the team used gaze direction, measured as the patients were having brain scans.
They then modeled the impact of hypothetical drugs to detect the level of treatment effect needed for detection by traditional statistical methods and machine learning.
“Stroke trials tend to use relatively few, crude variables, such as the size of the lesion, ignoring whether the lesion is centered on a critical area or at the edge of it,” said Tianbo Xu, the study’s first author.
“Our algorithm learned the entire pattern of damage across the brain instead, employing thousands of variables at high anatomical resolution. By illuminating the complex relationship between anatomy and clinical outcome, it enabled us to detect therapeutic effects with far greater sensitivity than conventional techniques.”
Just as they had suspected, the machine learning approach — taking into account the entire complexity of the damage — turned out to be superior. The advantage was particularly noticeable for drugs that reduced lesion volume.
Using traditional methods, a lesion would need to shrink by 78.4 percent for tests to detect the effect. The machine learning technique, meanwhile, required only a 55 percent shrinkage to detect a treatment effect.
“Conventional statistical models will miss an effect even if the drug typically reduces the size of the lesion by half or more, simply because the complexity of the brain’s functional anatomy — when left unaccounted for — introduces so much individual variability in measured clinical outcomes,” said Nachev.
“Yet, saving 50 percent of the affected brain area is meaningful even if it doesn’t have a clear impact on behavior. There’s no such thing as redundant brain,” he added.
The team now hopes that machine learning approaches — particularly valuable for studying complex systems such as the human brain — will find their way to clinical trials and other medical research settings.
“The real value of machine learning lies not so much in automating things we find easy to do naturally, but formalizing very complex decisions. Machine learning can combine the intuitive flexibility of a clinician with the formality of the statistics that drive evidence-based medicine,” Nachev concluded.